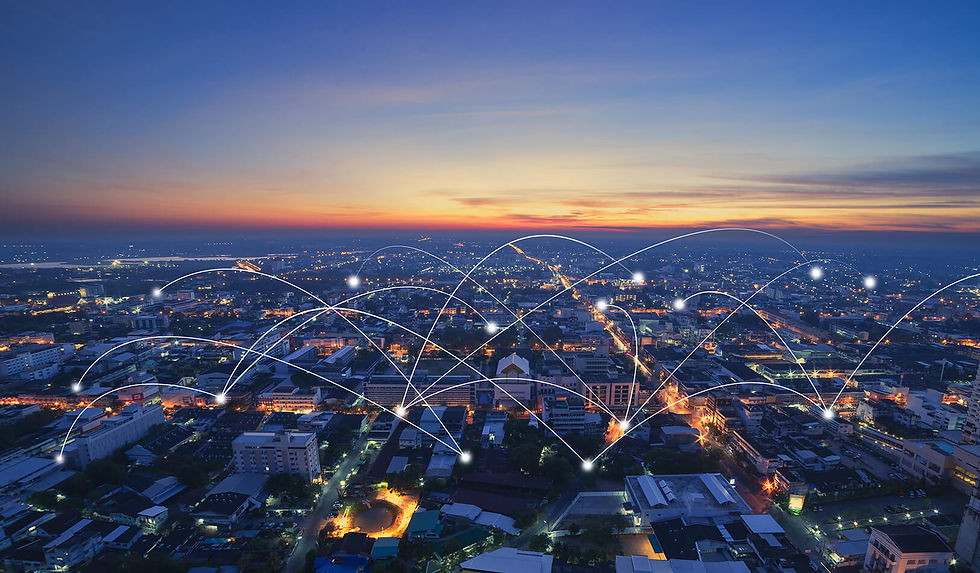
What is Mobile Network Data?
Most people in the UK possess a mobile phone and carry it around with them.
These phones communicate constantly with mobile phone networks, through cell towers, providing information about where those people are going from and to, their routes and speeds. This information, available on both a real-time and historic basis, provides a rich source of data for decision-makers across a range of sectors.
Whether you need to design a new town, predict train occupancy or identify how a housing development will affect local infrastructure, mobile network data (MND) will help you plan with confidence.
The Ultimate Guide to Mobile Network Data
Everything you need to know about using MND to understand how people move in urban areas
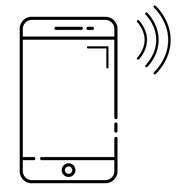
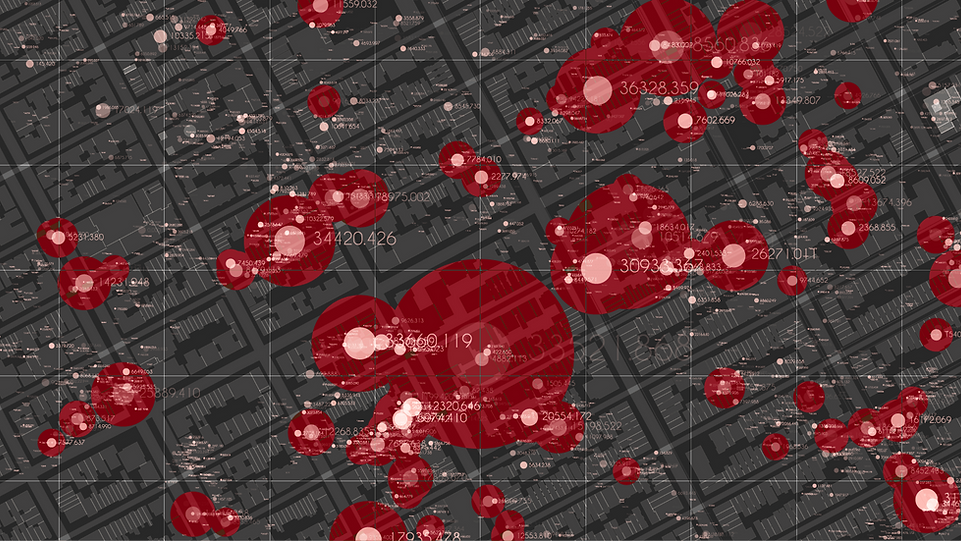
1. What is Mobile Network Data?
An invisible network of sensors
Mobile network data is the largest sensor network in the UK that nobody knows about.
Every mobile device acts as a sensor, providing information on its owner’s movements. If you wanted to create something similar for a business or local authority, it would take a considerable amount of time, resource and budget.
By contrast, mobile network data exists already and involves no additional maintenance or infrastructure.
2. The History of Mobile Network Data
Since 2015, MND has played a critical role in transport and urban planning
Any major transport or urban planning infrastructure project requires a large-scale capital investment programme, usually by local, regional and central government.
To ensure that money is being spent appropriately, data needs to be collected to create an evidence base for the project. This data provides the answers to questions such as why is the project needed, what its impact is likely to be and where it should be located.
Before the advent of MND, the main means of collecting data was roadside interviews. The outcome of that was a relatively small sample amount of data, which was extrapolated to show how people moved through a city. The data was limited, difficult and costly to obtain and required multiple resources including those of the police, who were needed to stop cars for the interviews. The market was ripe for disruptive technology.
To complement roadside interviews, transport and urban planners could use the normal exchange of information between a mobile handset and the network via signalling protocols. In simple terms, a mobile network needs to know where you are to route calls and data to you. It does not need to know exactly where you are, but to within the area of a mobile network cell.
This technology was initially developed in 2012 and was first used for a commercial purpose in 2015.
By developing advanced algorithms and applying them to the raw anonymised MND, a new product was developed for transport planners and engineering consultancies.
Initially, these groups were cautious. They were concerned about whether the insights that this data generated would be consistent with the existing understanding of what constituted normal behaviour in cities. They were also concerned about biases in the MND data and how these could be corrected as part of the processing and analysis of the data.
In fact, the mobile network data affirmed rather than undermined the previous findings about how people move around cities.
However, the volume of data was transformed. For the first time, planners were able to review tens or even hundreds of millions of journeys, yielding new insights and generating further data requests.
For example, in Liverpool, MND revealed that a large proportion of shopping traffic involved people trying to find car parks. When shoppers found a parking space, it was often on the wrong side of the city. The local authority used insights from MND to reduce congestion by enhancing the signage into the city centre to clarify the location of available parking spaces.
It became possible to carry out studies that would previously have been impossible, for example on motorways and A-roads where it would not be safe or practical to stop vehicles (cars, buses, coaches or HGVs) for interviews.
For transport planners, mobile network data became the ubiquitous data set for movement analytics.
It is now beginning to be used in other areas, such as airports, ports, HGV, freight and population movement.

3. Turning Mobile Network Data into Actionable Insights
Raw data is only the start of the process
Raw mobile network data itself won't give you actionable insights. It needs to be carefully filtered for the applicable geographic zones and timeframes, processed, mapped, expanded, aggregated and verified. It needs to be anonymised and GDPR compliant.
Once processed in this way, MND can provide insights into the way people move on foot, in a vehicle or by train.
By identifying and analysing demand activity in innovative ways, MND can be used to tackle complex spatial challenges in the sectors of Transport, Intelligent Mobility, Smarter Cities, Retail & Advertising, and the Built Environment.
The emergence of predictive analytics has coincided with an increasing recognition across UK plc and within the public sector of the value of the data and the insights that it can provide.
A recent Ofcom report confirmed that, in the UK, a third of internet users see their smartphone as the most important device for going online. With more than two thirds of people now owning a smartphone and using it for nearly two hours every day, it is becoming the hub of our daily lives.
Using mobile network data to capture movement patterns means that analyses can be based on a much larger sample size than traditional survey methods. It can also be combined with other data, such as GPS data for example.
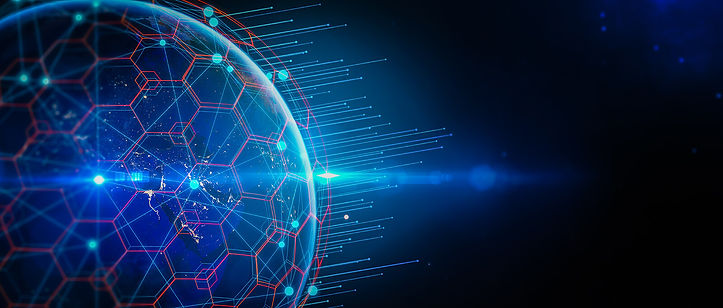
Need more information on how Mobile Network Data can support your project or strategy?
4. What can Mobile Network Data be used for?
MND has a huge range of potential applications
MND is particularly suitable for transport, urban planning, vehicle movement, population density, rail travel and airports.
Current applications include the following:
Transport Planning: Provide support to the development of transport models to test new developments, transport schemes, strategies and masterplans
City Planning: Design buildings and roads based on how people move around urban spaces
Rail: Estimate demand for services with real-time updates on train and station capacity and flow
Engineering: Gather clean and robust data to help engineering partners deliver successful projects, for utility services for example
HGV Transport: Understand routes and average speeds for greater efficiency and reduced environmental impact
Park & Ride: Support the selection of future locations based on insights into commuters' travel preferences
EV Usage: Understand where to install EV points based on where and when people charge their cars
Congestion Management: Minimise congestion and pollution by analysing traffic flow patterns
Event Planning: Make data-driven decisions on timing, road closures, diversions and staffing levels
Outdoor Advertising: Analyse population movement to establish prime locations for billboards and other formats.
Further use cases that are currently being explored include geofencing football grounds to gain a deeper understanding of the behaviour, origin and destination of football crowds. Using MND for further insights into theme park visitors is also under consideration.
Strengths and Limitations
MND is good for scale and giving the big picture. Using MND, 10 million observations can be collected in two weeks from a known sample, making it possible to extrapolate trips to full population movements, whereas GPS can record 1 million observations in a year from an unknown sample.
MND is less suited for studies where accuracy is more important than the sample size. It offers accuracy of up to 50m in urban areas, whereas GPS provides an accuracy of around 5m. However, it is possible to blend the two, for example, to calculate speed. Indeed, MND is often combined with GPS and other datasets.
a. Origin and Destination - People Movement
Where do people travel from and to?
Understanding where people are travelling from can provide insights into commuting, residential areas and shopping. Routes tell you a lot about the purpose of a journey and what services people are likely to need or want on their way from A to B, whether these are shops, petrol stations or electric vehicle charging points
MND helps to form a picture of people’s movements over time. From where their phone resides at night and during the day, it is possible to infer their home and work locations. It is best to get four weeks’ worth of data for transport planning. The more data a person has, the more value they can extract from the data.
For rail, trips can be split into ‘station to station’ and ‘first mile/last mile’ legs. This is helpful for firms and public sector bodies planning station upgrades and coordinating different modes of transport or planning of intermodal passenger transport.
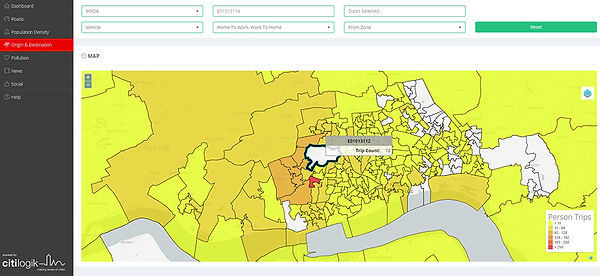
Understand how people move around your area with origin/destination (OD) information derived from Mobile Network Data.
b. Population Density
Where are people now at any given time?
MND can be used to view historic and near-real-time dynamic population counts to understand the number of people in and around a city for any given time.
This is invaluable for planning for large-scale gatherings such as New Year's Eve and Hogmanay, pop concerts, football matches, protests or demonstrations. Armed with the data, local authorities and businesses can make data-driven decisions on timing, road closures, diversions and staffing levels.

View dynamic population density changes in real-time across your city. Also view by static, slow-moving or fast-moving populations (traffic).
c. Mode of Transport
By what mode do people travel?
Modes of transport can be split into motorised, which comprises car, LGV, HGV, bus, motorcycle and rail, and active trips, which consist of walkers and cyclists.
How can MND distinguish between different modes of transport? Complex clustering algorithms consider speed, distance travelled and path for different land use contexts to separate motorised from active trips.
It is possible to distinguish rail journeys from other motorised trips by analysing the frequency with which devices interact with cells around the rail network and train stations. This is cross-referenced with train timetables to identify which train(s) passengers are taking. As well as the first and last stations, MND can calculate access/egress trips and station catchment areas by seeing where people leave home and go after leaving the station at their destination.
Further developments allow the identification of trucks (HGV) looking at long-term trend analysis and behaviour on the road network in terms of routing and speed.
As well as detecting the mode of each trip, algorithms can also identify how many people are stationary (such as present and staying in an area even if moving).
d. Journey Purpose
Why are people travelling?
Discovering the purpose behind a journey is crucial. With this in mind, you can differentiate between commuters and visitors.
For example, a well-known chain of roadside restaurants built a series of stores by busy roads, only to find that they were not attracting footfall because the majority of the traffic was local rather than people travelling long distances, who would be more likely to want to break up their journey with. a meal or a comfort break. Insights into the purpose of passing traffic would have enabled them to make better decisions about the location of their stores.
It is also possible to analyse the day and night-time locations to understand whether a person is at home or at work, although this distinction has become less clear-cut with the rise of working from home due to the Covid-19 pandemic.
e. Transportation Routes
What routes are people taking?
Understanding routes can help with analysing emissions, reducing congestion, the planning of new infrastructure and input into the Government's decarbonisation agenda.
MND can be used to snap the trajectories of each trip to the corresponding road or rail route. A ‘snap to flight’ offering is currently being developed for the aviation sector.
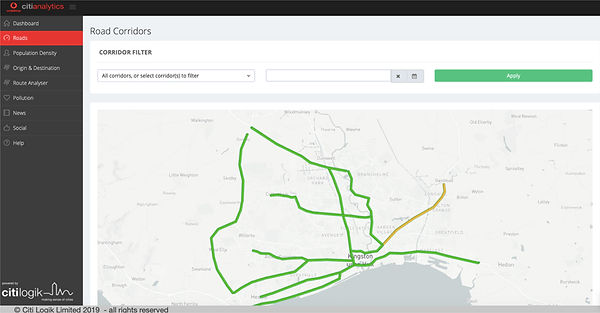
Monitor the performance of key corridors in your city in real-time with traffic state predictions, journey times, speeds and RAG indicators for current road performance.
f. Real-time and Historic Travel Trends
What are the travel patterns at different times or locations?
Real-time and historic travel trends are valuable in a range of contexts. For example, real-time data can help transport planners and users alike plan their journeys and ensure that delays on one mode of transport do not prevent travellers from making their connections.
Read about how real-time data has been used to accurately forecast train occupancy.
Historic trends are useful in understanding how travel behaviour has changed over a period of time, in response to specific situations such as maintenance works and accidents, and how it is likely to evolve in the future. These trends can include traffic volumes, speeds and journey times.
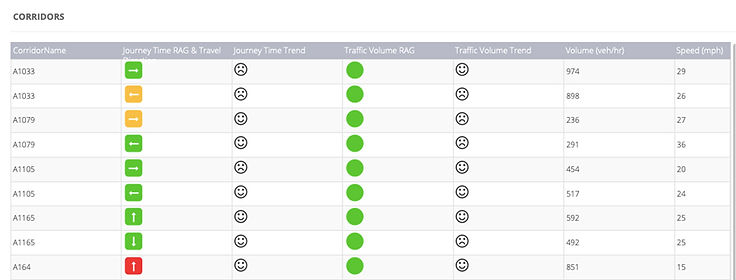
View traffic state information by corridor in real-time or review historic travel trends.
g. Pollution Reporting
What is the impact of travel on air quality?
MND can also be used to monitor travel movements and then be combined with sensors to assist with pollution reporting. Local authorities can use MND to plan and monitor traffic and transport in real time to minimise congestion and pollution. by informing the public of alternative routes using Variable Message Signs or other facilities.

View pollution emission information in real-time. Combine it with population density to understand pollution exposure and with traffic information to assess the impact of congestion.
h. Occupancy
How many people are occupying trains, stations or venues?
MND can monitor the usage of stations without reference to ticket sales and rail journeys. It is often possible to use a web portal to display the occupancy level within a station in real-time. MND is used to understand movement into and out of a station. This approach removes anyone who is within the area over extended periods, such as station and retail staff and people living and working next to the station.
Read about how MND enabled Govia Thameslink to collect real-time data about their trains on the East Croydon to Brighton line and use a simple traffic light system to identify which trains had seats available, which had standing room and which were full.

View train occupancy information in real-time.
i. The Impact of Delay
How much disruption will there be as a result of delays?
Following a major event that causes a delay, transport firms and planners often engage in what-if scenarios. They like to analyse after the event to learn lessons and work out how to improve things in the future. Armed with historic data, including origin destination, routing, and travel times, they are in a far better position to predict customer behaviour on the basis of how people have reacted in the past.
j. Travel Forecasting
How much traffic will there be and where?
Traffic forecasting has become sharply in focus due to the changing commuter behaviour brought about by the pandemic. The mode of transport and the times commuters choose to travel are expected to change, at least for a period of time. Changes in commuter behaviour have triggered changes in how transport planners and operators approach their work.
Planners have sought to delay updating their models and do not want to use data from 2019 as they are waiting to see what the new normal will look like.
However, waiting for a return to normality is not without risks. The risk is that planners use outdated models. Relying on existing data to make decisions about roadworks, highway planning, traffic flows and even cycle lanes could prove to be costly and statistically unreliable. Understanding the dynamics of how a city lives and moves in the new world is a whole new challenge.
Robust longitudinal data, like MND, can give transport planners and local authorities the evidence they need to win the support of residents and other stakeholders. With the right data, councils can easily explain and prove to their residents why they are making certain decisions or changes around road usage, which can prove especially useful when such decisions are controversial.
MND data can also offer the opportunity to undertake short term forecasting by looking at and extrapolating recent changes in travel behaviour to produce predictions of demand in minutes, days or months.
Read about how Hull City Council used real-time passenger traffic data to help shape the road network and parking facilities while easing congestion.
k. Location Planning
Where are the best locations for roads, housing developments and services?
By understanding the movements of people and traffic, MND can offer invaluable insights into the best locations for everything from residential or business developments to Park & Ride facilities to electric vehicle charging points. Such facilities need to be carefully located and must leverage existing commuter behaviour patterns to be effective. For electric vehicles, optimal locations for charging points are based on traffic volume, which can be identified, categorised and counted in a non-disruptive manner.
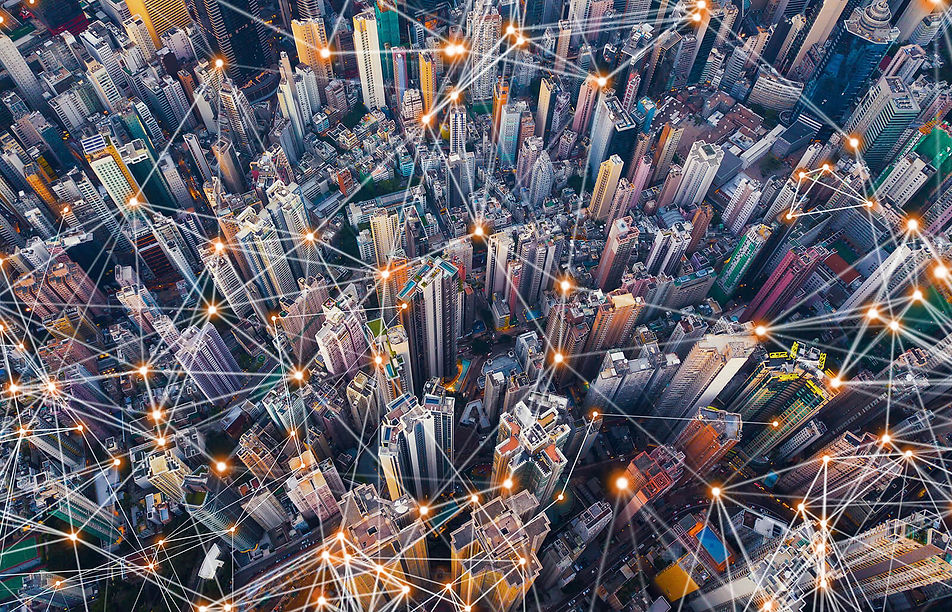
5. Mobile Network Data Applied
How do Mobile Network Data analytics fit into management and planning?
City and transport planners, operators and policymakers need to understand the interplay of road infrastructure, travel patterns, travel times and congestion. This is critical to plan work patterns, public transport operations and urban regeneration against a backdrop of rising populations and urbanisation - two trends exerting increasing pressure on cities' infrastructure.
Advanced demand estimation models incorporating big data sources including mobile network data can provide these insights, helping to guide decision-makers on investments in road infrastructure construction, maintenance and transport operations.
It is far cheaper to buy MND than to install infrastructure in the wrong place. It is also easier to justify decisions armed with the facts and figures to show the effect that a transport or urban development is going to have or has had in the past.
a. Urban Planning
How is Mobile Network Data used to help with city planning projects?
Mobile network data enables city planners to understand congestion, current and predicted journey time across key routes, people and pollution to make informed future planning decisions. This can help with traffic management, planning major events and emissions tracking.
MND offers historic and real-time insights into roads, origin-destination and routes, such as:
-
Foot passenger movement to and from train/underground stations for retail planning, pedestrian planning (i.e. crossing busy roads)
-
In the development of sites of interest e.g. stadiums, new tourist attractions for planning and forecasting of future travel movement to and from the destination
-
Planning around major events such as concerts and sporting events
-
The impact of pollution control measures on traffic in key urban areas
-
Footfall in an area including night time economy
b. Rail
How is Mobile Network Data used in rail projects?
Train operating companies (TOCs) need to understand train occupancy and people flow to make quick and well-informed decisions in areas such as timetabling, health & safety, maintenance planning and response to incidents.
MND can provide the insights required either in real-time or as historical data files. These insights include railway station catchment data, impacts of train delays, station crowding analytics and the impact of Covid-19.
Potential uses include the following:
-
Real-time and historic occupancy
-
Passenger flow at stations
-
Railway station catchment data
-
Impacts of train delays
-
Station crowding analytics
-
Impact of Covid-19
c. Civil Engineering
How is Mobile Network Data used in civil engineering projects?
MND enables engineering companies to deliver successful data-driven projects for local authorities, where these focus on transport projects, urban planning or trade flows.
Insights include movements of people, including origin and destination and rail station occupancy, These can be critical to decide on the best options for the routing and timing of construction traffic and for when to undertake the work.
d. Transport Planning
How is Mobile Network Data used in transport planning projects?
MND can be applied to every aspect of transport planning. It can identify when and why people are travelling, what mode of transport people are using, their routes and speeds on a real-time and historic basis. This is invaluable in planning new roads, train timetables, traffic management and easing congestion.
e. HGV Movement / Trade Planning
How is Mobile Network Data used in transport and logistics?
MND can identify HGVs, enabling hauliers and local authorities to make data-driven decisions on logistics, traffic and routes to optimise efficiency, reduce fuel consumption and increase profits.
It can provide insights into HGV routes, origin-destination, dwell time, volume, delays and border-crossing.
To learn about how mobile network data helped the Department for the Economy in Northern Ireland plan for Brexit.
f. Electric Vehicle (EV) Planning
How is Mobile Network Data used in planning for electric vehicles?
Electric Vehicles are set to transform our roads, but gathering data about usage patterns has historically been challenging.
MND can categorise and count traffic volumes in a non-disruptive manner and give insights into origin-destination, optimal locations for charging points based on traffic volume, distance travelled, relation to home and work, and identify peak and off-peak periods to monitor infrastructure impacts.
For EV manufacturers, MND can offer greater insight into consumers to help with decision-making. It can help the public sector interpret EV travel patterns and the impact on infrastructure and traffic management, while for forecourt firms MND can identify the most financially viable charging locations.
g. Park & Ride
How is Mobile Network Data used for Park & Ride projects?
Park & Ride services need to be carefully located and must leverage existing commuter behaviour patterns to be effective. MND enables planners to locate services based on traffic movements and to understand the origins and destinations of potential users.
It can offer insights into potential passengers driving past Park & Ride locations, the origin-destination of commuting traffic, the optimal location of new sites and the potential for the provision of EV charging points.
h. Housing Developments
How is Mobile Network data used in development planning?
Mobile Network Data can help identify sites where new housing can be built to meet growing demand. MND gives an accurate picture of how people move around urban areas, including their origins and destinations.
To read more about how MND can inform future housing planning, read how Citi Logik advised Dorset County Council in their projects.

6. The Future for Mobile Network Data
Conclusion
We hope that this page has given you a greater understanding of the applications of Mobile Network Data in the public and private sectors.
The use of anonymised mobile network data (MND) to understand mobility patterns has become increasingly popular. In just five years, it has become the ubiquitous data set in transport and urban planning. Traditional survey methods are expensive, potentially disruptive, prone to human error and provide only a limited snapshot in time.
MND is considerably more cost-effective and can provide information on population and vehicle flows both historically or in real-time. MND has a valuable role as an integral data source providing insights to better inform decision making in the development of transport and urban planning models.
MND has a huge amount of untapped potential. Many people have never heard of it or seen it in action. In the future, it could be applied to a far broader range of sectors in the future, as well as being combined with emerging technologies such as the Internet of Things.
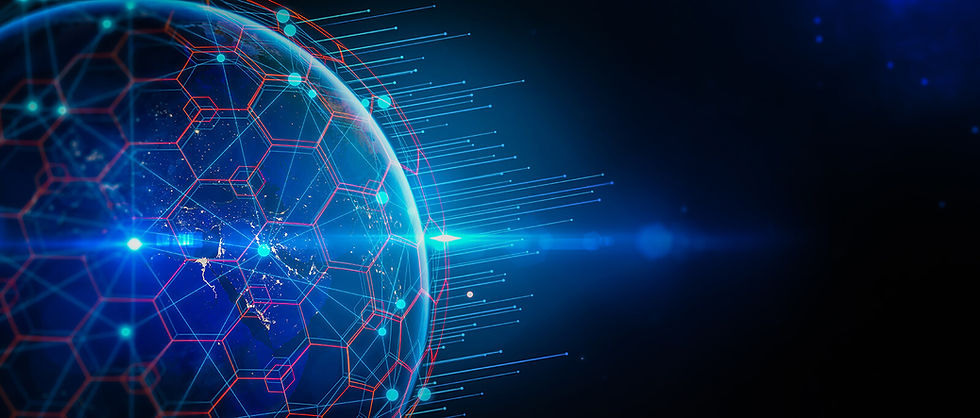
CONTACT US
Get in touch with us to discuss how Mobile Network Data could help your project
Please contact us to discuss how our expertise in anonymised mobile network data can provide you with critical insight to inform your projects and planning strategy.
We are happy to provide testimonials and further details on case studies on request.